Connectionism, A Model Of Neural Functioning
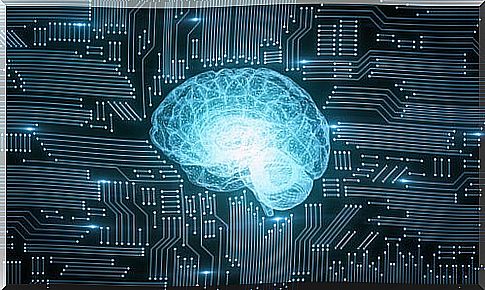
Understanding how the brain works is one of the greatest challenges facing psychology. Hence the existence of the various approaches and perspectives. In fact, after the appearance of cognitive psychology and the Turing machine there was a revolution in this field. From this moment on, the brain began to be viewed as an information processor.
The first theory that was created to explain the workings of the brain was the computational metaphor, but it soon began to have flaws. Taking this situation into account, cognitive psychologists, with the intention of looking for new explanations, created a theory known as connectionism.
However, before explaining what connectionism is, it is important to understand the view of cognitive psychology on the brain. In this way, we will understand the implications and flaws of the computational metaphor. For this reason, we will review the main aspects of this branch of psychology in the next section.
Cognitive psychology and the computational metaphor
Cognitive psychology understands the human brain as an information processor. This means that it is a system that is capable of coding the data from its environment, modifying it and extracting new information from it. Furthermore, this new data is incorporated into the system in a continuum of inputs and outputs .
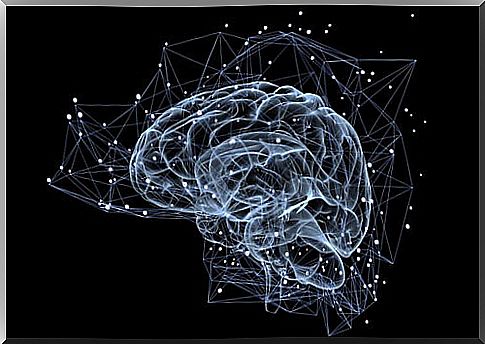
The computational metaphor explains that the brain is like a computer. Through a series of programmed algorithms, it transforms the information inputs into a series of outputs . This may at first seem to make sense, since we can study some human behaviors that fit this model. Now, if we explore a little more, we begin to detect flaws in this perspective.
The most relevant errors are the speed with which we process the information, the flexibility with which we act and the imprecision of our answers. If our brain had programmed algorithms, we would have responses of another type: slower due to all the processing steps to be carried out, more rigid and much more precise than they are. In short, we would be like computers, and with the naked eye we see that this is not the case.
Although we can make attempts to adapt this theory to the new evidence, changing the rigidity of the programmed algorithms for others that are more flexible and capable of learning, we would still continue to identify flaws in the computational metaphor. And this is where connectionism comes in , a current that is simpler than the previous one, and that explains the brain’s information processing in a more satisfactory way.
What is connectionism?
Connectionism leaves behind computational algorithms and explains that information is processed through propagation patterns of activation. But what are these patterns? In simpler language, this means that when an input of information enters your brain, the neurons begin to activate forming a specific pattern, which will produce a specific output. This will form networks between neurons that will process information quickly and without the need for pre-programmed algorithms.
To understand this, let’s put a simple example. Imagine that a person tells you to define what a dog is. When the word reaches your ear, the set of neurons associated with it will automatically be activated in your brain. The activation of this group of cells will spread to others with which it is connected, such as those related to the words mammal , bark or hair . And this will activate a pattern in which these characteristics are included, which will lead you to define a dog as ‘a mammal with hair that barks’.
Properties of connectionist systems
According to this perspective, for these systems to work as the human brain seems to behave, they have to meet certain conditions. The basic properties that must be followed are the following:
- Activation propagation. This means that neurons, when activated, influence those with which they are connected. This can occur by facilitating its activation or by inhibiting it. In the example above, dog neurons facilitate mammalian neurons , but inhibit reptilian neurons .
- Neural learning. Learning and experience affect the connections between neurons. Thus, if we see many dogs that have hair, the connections between the neurons related to both concepts will be strengthened. This would be the way in which the neural networks that help us with processing would be created.
- Parallel processing. Obviously this is not a serial process, neurons are not being activated one after another. The activation propagates in parallel between all neurons. And it is not necessary to process one activation pattern after another, multiple can occur at the same time. Thanks to this we are able to interpret a large amount of data at the same time, although there is a limit to our capacity.
- Neural networks. The system would be a large network of neurons grouped together, through inhibition and activation mechanisms. Information inputs and behavioral outputs would also be found within these networks . These groupings would represent the structured information that the brain has, and the activation patterns would be the way in which the processing of said information occurs.
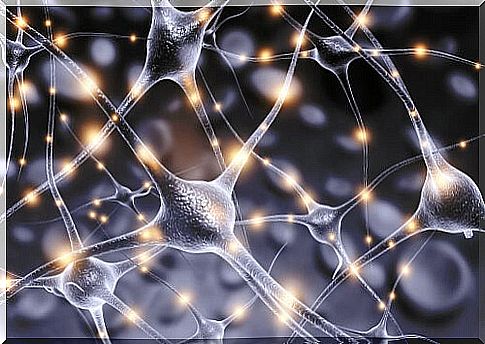
Conclusions
This way of interpreting neuronal functioning not only seems very interesting, but the studies around it seem fruitful. Today, computer simulations of connectionist systems have been created on memory and language, which closely resemble human behavior. However, we still cannot say that this is the exact way the brain works.
In addition, this model has not only helped to contribute to the study of psychology in all its fields. We also find multiple applications of these connectionist systems in computing. Above all, the theory has been a great advance in studies about artificial intelligence.
To conclude, it is important to understand that the complexity of connectionism is much greater than that raised in this article. Here we can find a simplified version of what it really is, useful only as an approximation. If your curiosity about the subject has been piqued, feel free to continue investigating this theory and its implications.